“I’m Sorry Dave, I’m Afraid I Can’t Do That.” What to Make of Synthetic Data?
Posted: 05/09/2024
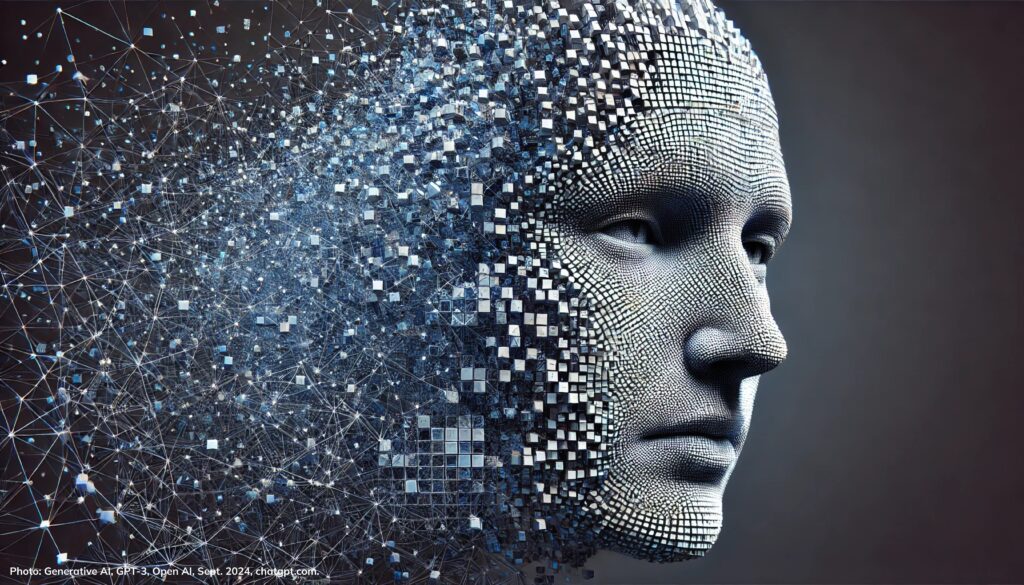
Summary
- Synthetic data is generated by Large Language Models with the aim of replicating human survey data, to be used when surveys are too expensive or difficult to run.
- The academic evidence for whether or not synthetic data can accurately replicate human responses is mixed and the debate is far from settled.
- While synthetic data can sometimes closely replicate human survey responses, it is likely to be least accurate in the cases where it would be most useful.
“I’m sorry Dave, I’m afraid I can’t do that.”
In recent months there has been a lot of discussion in the market research world1 about synthetic data: responses generated by artificial intelligence to mimic survey data collected from real humans. In a Marketing Week column late last year2, Professor Mark Ritson wrote about a recently published academic paper3 that used Large Language Models (LLMs) to generate perceptual maps of brands and compared them to human-generated data. His conclusion?
“The era of synthetic data is clearly upon us. This study is one of more than half a dozen that have been completed recently by elite marketing professors at elite business schools, each with similar jaw-dropping results and knee-wobbling implications. Most of the AI-derived consumer data, when triangulated, is coming in around 90% similar to data generated from primary human sources.”
Sounds wonderful, right? But do those claims stand up to scrutiny?
“It doesn’t matter what’s under the hood. The only thing that matters is who’s behind the wheel.”
Let’s start with the paper which sparked those claims. Professor Ritson presents two perceptual maps of car brands, one from LLM-generated data and the other from 530 human subjects (Figure 1). He states that “The professors use a very complex ‘triplet method’ to assess the congruence between synthetic and human data. They show that there is a 90% similarity between the two charts.” Actually, the paper states that “we observe an agreement rate 87.2% as high as the theoretical maximum of 94.7%”, which is a more modest (though still impressive) 83% similarity.
Figure 1 – Perceptual maps of automotive brands
Source: Peiyao Li, Noah Castelo, Zsolt Katona, Miklos Sarvary (2024) Frontiers: Determining the Validity of Large Language Models for Automated Perceptual Analysis. Marketing Science.
The authors go on to look at heterogeneity in the synthetic data, to assess whether they can replicate differences in consumer preference that correlate with attributes such as age, gender, or income. They conclude that the technology “may also allow to find qualitative effects of consumer heterogeneity … [but] … we do not recommend using the exact coefficients derived from LLM-generated data as the confidence intervals do not match the human data.” In other words, the results pointed in the right direction, but the estimated differences were very different from the human data. Worse, the authors also admit that “we have replicated our study in additional product contexts (namely, apparel brands and hotel brands). We found that, generally car brands perform better than apparel brands, which in turn are better than hotel brands.” It is at least refreshingly honest to admit to publication bias…
This pattern of results is exactly what one would expect, given how LLM models work and the data that they have been trained on. LLMs are built around embeddings: high-dimensional vectors describing the semantic relationship between words and phrases, learnt from large corpuses of online text. Most car brands have been around for decades, are found all over the world, and are discussed at length in many online articles and discussion forums. It therefore makes sense that the embedding would capture the semantic relationships between car brands as well as with various adjectives such as sporty or expensive. Reliable markers of characteristics of the authors of online opinions, such as the writer’s age or gender, are much less common so the embedding is less likely to capture such relationships. Brands in other markets are discussed less often than cars or are local rather than global, so the embedding simply doesn’t capture much information about those brands, if at all.
“Houston, we have a problem”
What about the wider claim that “most” AI-derived data is around 90% similar to human data? Here’s one example of those studies, Fell (2024)4, which attempts to replicate social surveys about energy with data generated by LLM “agents”. Figure 2 shows the original human survey results and the LLM-derived replication, and it is immediately obvious that the synthetic data is very different to the human survey responses. The author concludes that “The replication […] showed some similar trends to the original […] Statistical test results were partially replicated. However, some key observations from the original study were not apparent.” His conclusion is at odds with Professor Ritson: “it is far from possible to conclude that LLM-based agents could reliably replace human respondents at this time.”
Figure 2 – Consumer demand to participate in peer-to-peer energy trading
Source: Fell, Michael, Energy Social Surveys Replicated with Large Language Model Agents (January 6, 2024).
Other recent studies have found that “our work casts doubt on LLMs’ ability to simulate individual-level human behaviour across multiple-choice question answering tasks”5 or that “results highlight the pitfalls of using LLMs as human proxies”6. Of course, this is just a small sample of academic papers, but we found them by (you guessed it) asking Chat GPT to tell us about using synthetic data to replace human survey respondents.
“I’ll be back.”
In short, the hyperbolic claims about synthetic data are currently just that: hyperbole. In limited cases LLM-generated data can somewhat closely replicate human survey responses, but the nature of how LLMs operate and the data they are trained on means that synthetic data is likely to be least accurate in the cases where it would be most valuable: new brands, propositions, and markets or niche and hard-to-reach audiences. In the future, larger models trained on even more data may start to reduce the gap between synthetic and human data, but we are currently very far from “the era of synthetic data”.
Finally, just a Dectech reminder that both synthetic and human survey data are often not much use for predicting how consumers will actually behave. Traditional survey approaches are based on unrealistic assumptions of rationality and ignore the importance of contextual factors and constructed preferences in determining choices (see for instance Gal and Simonson (2020)7 for more on that topic). That’s why we developed BehaviourLab8, our immersive behavioural experiments for making accurate forecasts of how people will behave in new situations. Of course, using real human respondents!
1. E.g. https://www.mrs.org.uk/campaign/id/synthetic?MKTG=SYNTHETIC.
2. https://www.marketingweek.com/synthetic-data-market-research/.
3. Peiyao Li, Noah Castelo, Zsolt Katona, Miklos Sarvary (2024) Frontiers: Determining the Validity of Large Language Models for Automated Perceptual Analysis. Marketing Science.
4. Fell, Michael, Energy Social Surveys Replicated with Large Language Model Agents (January 6, 2024). Available at SSRN: https://ssrn.com/abstract=4686345 or http://dx.doi.org/10.2139/ssrn.4686345.
5. Petrov, N. B., Serapio-García, G., & Rentfrow, J. (2024). Limited Ability of LLMs to Simulate Human Psychological Behaviours: a Psychometric Analysis. arXiv preprint arXiv:2405.07248.
6. “Do LLMs Exhibit Human-like Response Biases? A Case Study in Survey Design” (Tjuatja et al., 2023).
7. Gal, David & Simonson, Itamar. (2020). Predicting consumers’ choices in the age of the internet, AI, and almost perfect tracking: Some things change, the key challenges do not. Consumer Psychology Review. 4. 10.1002/arcp.1068.
8. https://www.dectech.co.uk/behaviourlab/.