Benny Cheung on the Brainy Business podcast
Insurance Fraud Prevention
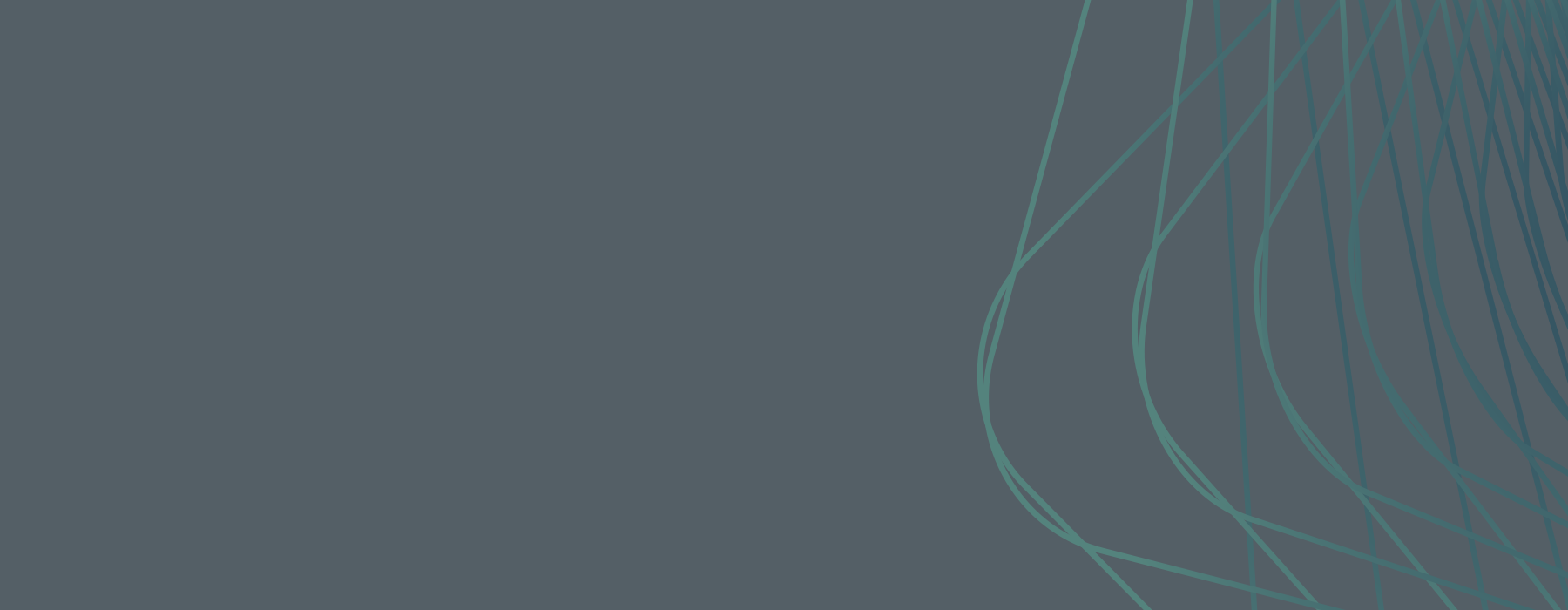
See below for additional information on the case study
Crafting behavioural Nudges
Tacking retail opportunistic insurance fraud is a difficult task as the fraudsters often think it is a victimless crime and act on false beliefs. By understanding the point at which the problem occurred (the behaviour) and the underlying cause (the beliefs), a behavioural framework could be constructed to approach tackling the problem: if you can change the beliefs then you can change the behaviour. In order to change the beliefs it was proposed that messages be created to address these beliefs directly, as well as apply principles from behavioural science, and then to apply the messages in the operational communications in the form of subtle behavioural ‘nudges’ in claims and applications processes.
To devise the behavioural ‘nudges’ to use, an extensive review of academic literature was carried out of principles for influencing behaviour, as well as considering how these may apply to alter the individual beliefs that may be held. For example, the belief that “everyone is doing it” relies on the principle of ‘norming’ – people seek to behave like their peers, so if “everyone else is doing it” then they will too. In this instance a simple message explaining that most people are honest and accurate when completing claims or applications may help to change the erroneous belief. In total, 18 messages were created spanning five principles. These principles, as well as example applications, may be seen in the table below.
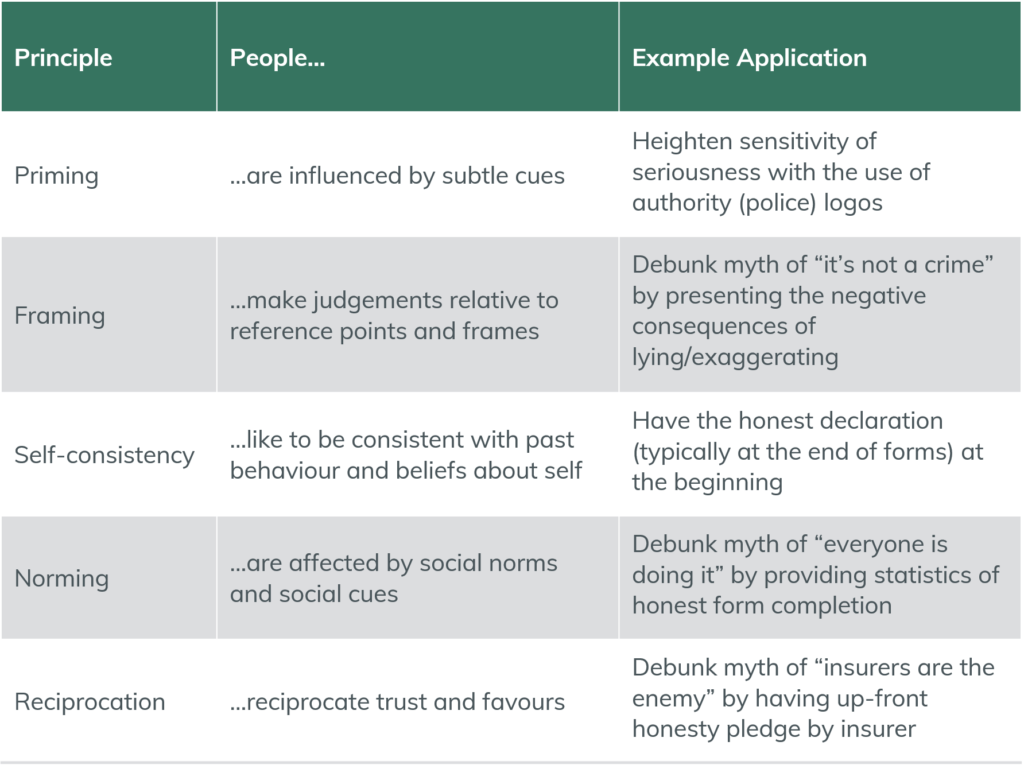
Experiment Paradigm
We believe that recreating a realistic journey is crucial to making reliable predictions about customer behaviour. To test the effectiveness of the designed behavioural ‘nudges’, we asked participants to complete a simulated online motor insurance application or claims process (participants were randomly assigned to one or the other). Participants were presented with a scenario explaining that they were either making an application or a claim (for the latter they were asked to imagine their car had been broken into, with approximately £1,000 worth of items stolen), and asked to fill in the online form as they would in real life. In both cases, the online forms that participants went through were designed to replicate real-world forms.
In order to test the impact of any messaging it was important for the online forms to include questions that participants might lie or exaggerate to in order to get a better deal for themselves (the ‘contentious question’). The contentious question needed to be one that could realistically be asked in an online application or claims form, but also one that related to a reasonably high prevalence behaviour. The question “have you ever been caught speeding?” was therefore chosen, and included in the forms and presented along with a series of other questions so as not to stand out. An operational comms message (the ‘nudge’) was then presented as a pop-up message just before this series of questions (with no pop-up message in the control condition).
Another crucial aspect of the research design was to understand the genuine underlying prevalence of speeding so that the rate of lying in the experiment could be understood. In order to do this the Unmatched Count Technique (UCT) was used. The UCT works by taking two samples and presenting one of them with a set of statements and asking them simply how many of them are true of them (e.g., “I have been to France”, “I ate a chocolate bar today”, etc). The second sample is presented with exactly the same task and statements, except with the addition of the ‘contentious’ statement of interest (in the case of this research this was the statement “I have been caught speeding”). With some simple maths, comparing the means of the two samples, the prevalence of the contentious statement being true may be calculated. Peter Kellner, founder of the YouGov opinion polling organisation, did a brilliant job explaining this technique in this article.
By understanding the underlying prevalence of speeding, the proportion of people lying in the control condition (the standard application or claims process outlined above, with no interventions included) could be calculated. In turn, the impact of any intervention in reducing the rate lying could be calculated by comparing it to these figures.
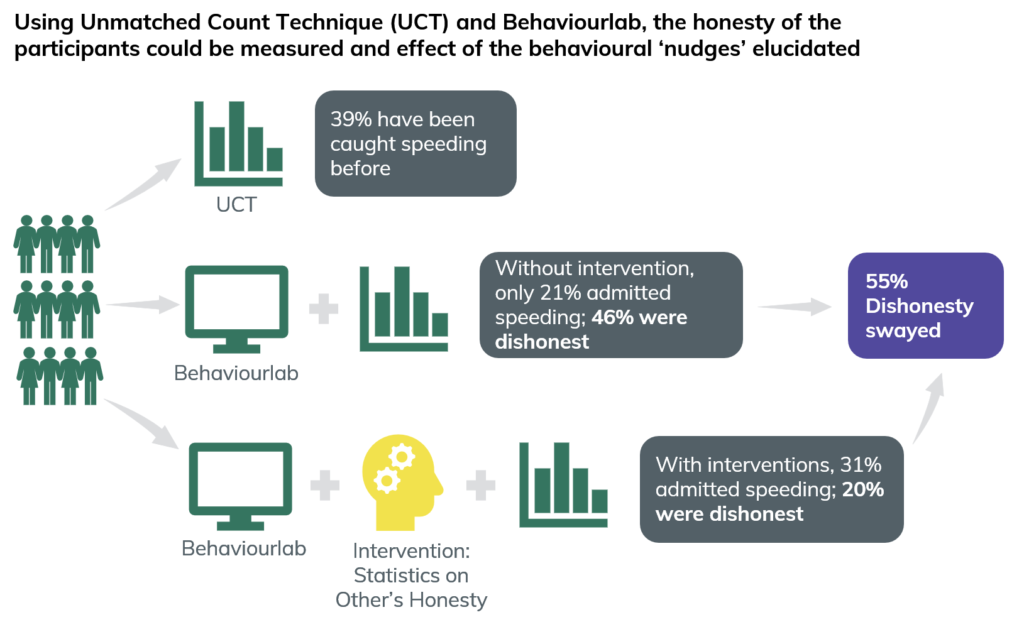
Subscribe to receive our latest insights and news on insurance fraud prevention
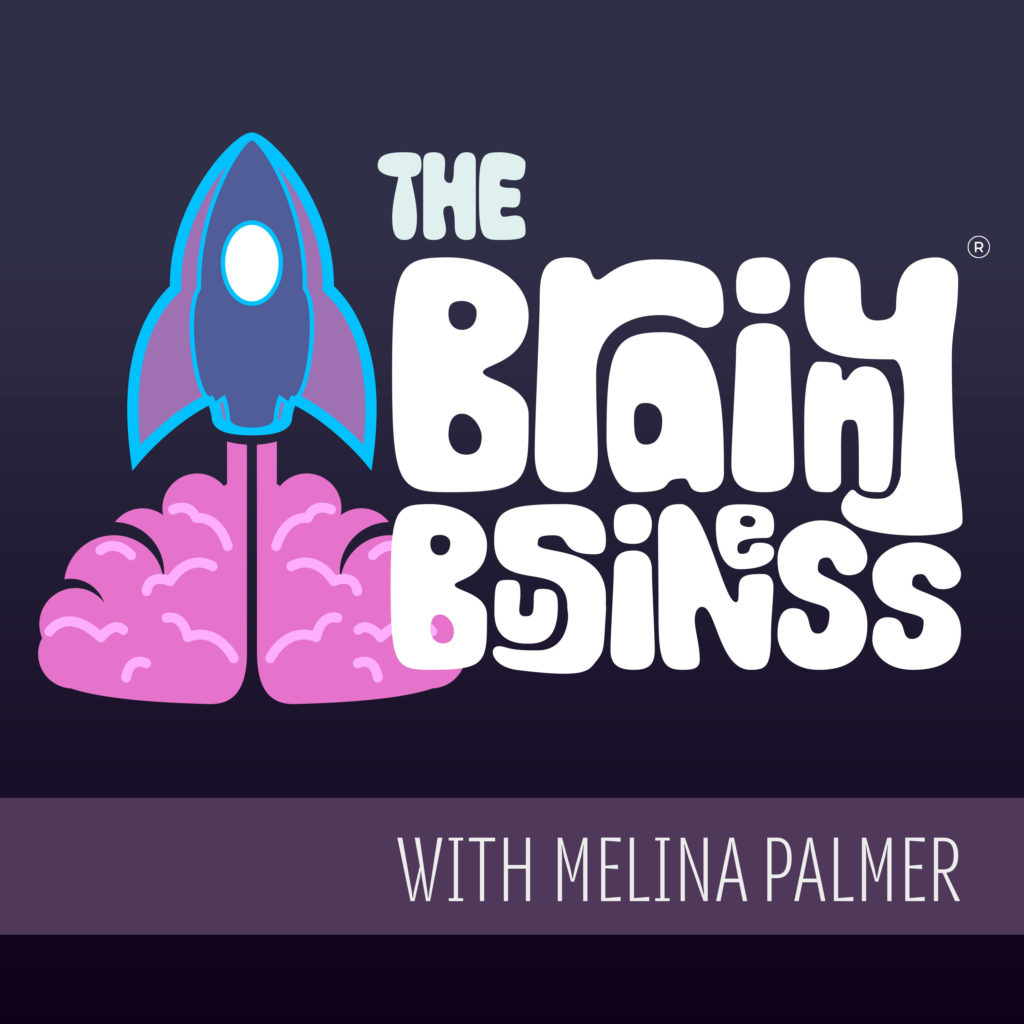
Tackling Opportunistic Insurance Fraud Animation
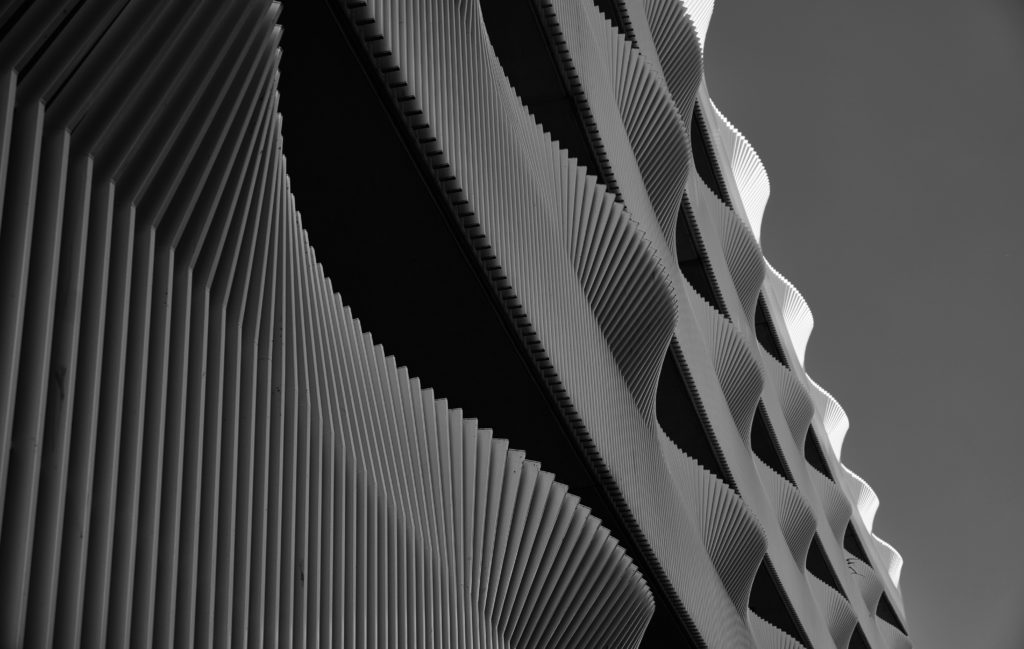
Dectech win MRS Award for Financial Services Research 2020
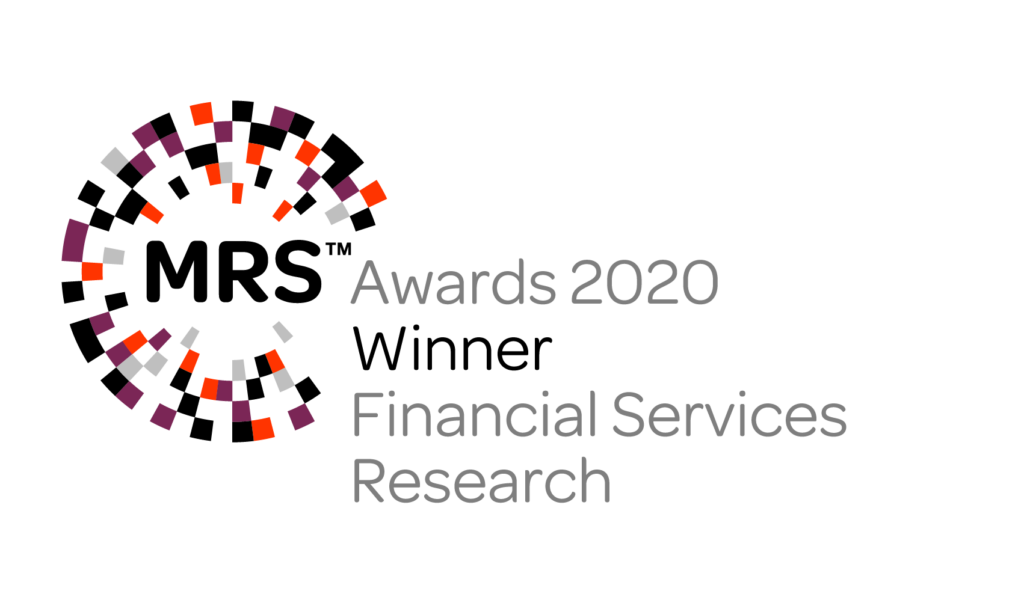
Benny Cheung in a Podcast with Human Risk
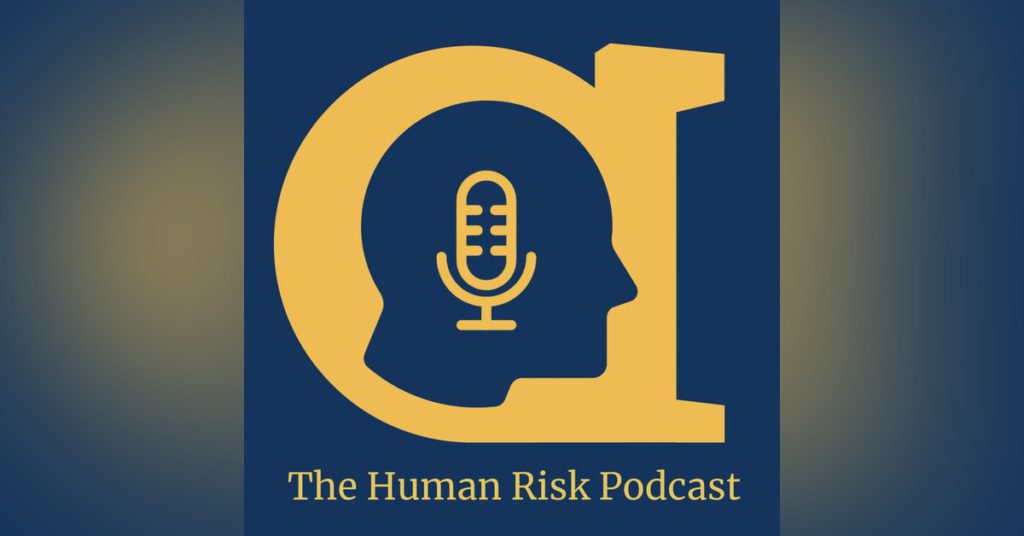
Dectech is the finalist in the Outsourced Partner of the Year category for the British Claims Awards 2020
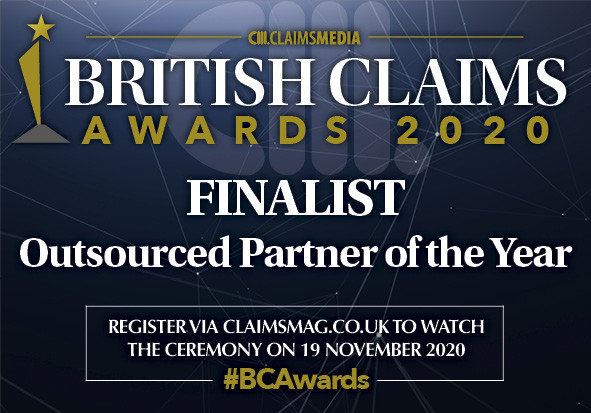
Dectech Director Benny Cheung speaks at the Behavioural Science Summit 2020
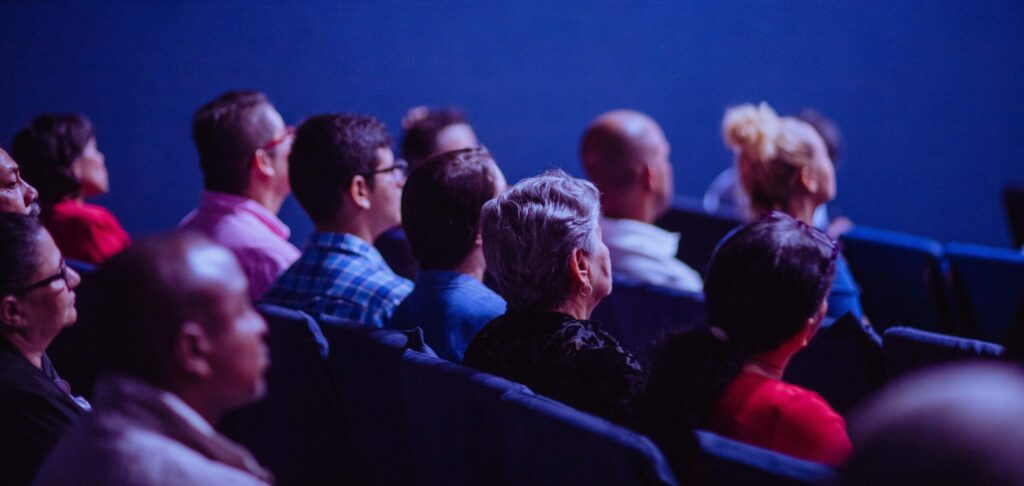